|
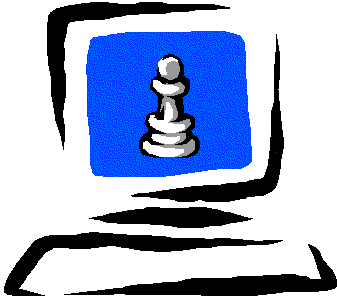
About the Chessmetrics Rating System
|
There are already two widely-accepted rating systems in place: the official FIDE ratings and the Professional ratings. The FIDE ratings have been calculated yearly since the early 1970's, twice a year starting in 1980, and now four times a year starting in late 2000. Before 1970, the only widely-known historical ratings are those calculated by Arpad Elo, the inventor of the Elo ratings system used by FIDE. These historical ratings, which appeared in Elo's 1978 book The Rating of Chessplayers Past & Present, were calculated every five years, using only games among top-80 players within each five-year-span, and Elo only reported on the best of these ratings ever achieved by each player. With the exception of a tantalizing graph which displayed the progression of ratings (every five years) for 36 different players, there was no way to see more than one single rating for each player's entire career.
Elo's historical rating calculations were clearly an incredible accomplishment, especially considering the lack of computational power available to him more than two decades ago. However, with modern game databases, better computers, and more than two decades of rated games to indicate how well the FIDE ratings work, it is just as clear that the time is long past overdue for the next generation of historical ratings. In the past year, it has gradually become clear to me that I should be the one to calculate those ratings. Once I reached the decision to move forward with this project, there were three big questions to answer:
(1) How far back in time should I go? That one is pretty easy to answer. The first international chess tournament was held in London 1851, and before that time most recorded games are either individual matches or casual games. Starting in 1851, there are increasingly more recorded games available, but there were still enough games in the pre-1851 era to allow for an initial pool of rated players to be built, based on games played between the start of 1830 and then end of 1850. Once that initial pool was built, it became possible to start calculating yearly ratings, with the first rating list appearing as of December 31st, 1851.
(2) Where should the raw data come from? The first time I tried to do historical ratings, in early 2001, I used the only large game collection I owned, which was the Master Chess 2000 CD. To supplement it with games right up to the present, I used games downloaded from the TWIC (The Week in Chess) site. The result was the ratings which have appeared on the Chessmetrics site for the past several months. Unfortunately, there was no standardization of the spelling of player names on the MC2000 CD, so I had to do a tremendous amount of manual work in standardizing them, so that Ratmir Kholmov (for instance) would show up as one person rather than five different people named "Holmov, R", "Kholmov, R", "Kholmov", "Holmov, Ratmir", and "Kholmov, Ratmir". I tried to do this accurately, but I'm sure there must have been errors. In addition, there does seem to be extensive duplication or omission of games. The results were nevertheless quite useful, but the feedback I got from readers led me to conclude that the ChessBase game collection CD's like MegaBase would work better, since many more games were included and there was much better (though not perfect) standardization of player names. I still had to go through the process of identifying players with multiple spellings, and cleaning up duplicate games, but it was definitely easier than before. The CD I bought only went through mid-2000, so I still had to supplement it with more recent games from TWIC.
(3) What formula should I use? When I did my first try at historical ratings, using the Master Chess 2000 games, I tried many different approaches, eventually settling on a compromise which combined three different approaches: a simultaneous iterative approach similar to how the initial pool was generated for the Professional ratings (and for my initial pool of players), a statistical "Most Likely Estimate" approach which used probability theory, and also the traditional Elo approach. I tried to use this compromise solution again on the ChessBase data, but eventually discarded it because it was taking too long to calculate, and I was also identifying some problems with how provisional players were entering the system. I decided to start over from scratch and see what I could do with the benefit of several months of experience in developing rating systems.
The obvious first step in calculating 150 years of retroactive historical ratings, based on the ChessBase games, would be to use the Elo formula itself. This is indeed the first thing that I did. Then I went back and applied the expected-score formulas to all historical games in my database, using those ratings, and compared the prediction with the actual results, to see how well the ratings worked at actually predicting the outcome of each historical game. I found significant deviations between the predicted and actual outcomes, suggesting that the Elo formula could itself be improved upon. After considerable statistical analysis of this data, I eventually arrived at a formula which seemed to work significantly better than the Elo scheme. I believe that in addition to working better than the Elo scheme, my Chessmetrics ratings have just as solid a grounding in statistical theory.
In order to calculate a player's Chessmetrics rating, we need to know what their rating was exactly a year ago, as well as their performance rating based on all their (rated) games that were played during the past year. The quantity of games played is also very important. If you played only a few games over the past year, then we are going to mostly believe your older rating, with only minor adjustments based on those few games. This is similar to how the FIDE ratings work, where you have an ongoing rating which gets changed a little bit after each game you play. The Professional ratings don't work very well in this scenario, since you have to go so far back in time to include the 100-most-recent games.
On the other hand, if you played a hundred games in the past year, then we don't really care too much what your rating was a year ago. There is so much evidence (from those hundred games) of your current level of play, that we can basically say that your recent "performance rating" (over that entire year) is the best estimate of your current level of play. This is similar to how the Professional ratings work, where a performance rating of your most-recent 100 games is calculated and becomes your new rating. The FIDE ratings don't work as well in this scenario, since the old rating is increasingly out-of-date when a player plays frequently, yet the old rating is still what is used for the ongoing rating calculations, until the next rating period. Even having more frequent FIDE calculations (now quarterly) doesn't help nearly as much as you would think.
Since most players' number of games per year will be somewhere in the middle, the best compromise is a combination of the two approaches, a rating formula that works equally well for frequent and infrequent players. Of course, it is also important to know whether that older rating was based on just a few games, or whether there was a lot of evidence to justify the accuracy of the older rating. For instance, if two years ago you were very active, then we can have a lot of confidence that your rating a year ago was a pretty good guess at your level of play at that time. On the other hand, if you played very infrequently two years ago, then we will place correspondingly less emphasis on the accuracy of that rating from a year ago, and even more emphasis on your recent results than we "normally" would.
You can think of a Chessmetrics rating as a weighted average between the player's rating a year ago, and the player's performance rating over the past year. The weights are determined by the accuracy of that year-old-rating (e.g., whether it was based on many games or few games) as well as the accuracy of the performance rating over the past year (e.g., whether it represents many games or few games). The ratings are era-corrected, anchored to a particular spot further down in the rating list (the specific spot is based on the world population; in 2001 the #30 player always gets a particular rating number, and everyone else is adjusted relative to that player), such that a 2800 rating should typically be about the level needed to become world champion.
Ultimately, it is up to each person to decide which rating system they trust most. To help you in this decision, please allow me to mention some of the advantages that the Chessmetrics ratings have over the FIDE and Professional ratings. To be fair, I will also mention all of the disadvantages that I am aware of, though you'll have to forgive me if I don't criticize my rating system too fervently.
The FIDE rating system has a serious drawback in that it is heavily dependent on how frequently ratings are calculated. For the same set of games, starting from the same initial ratings for everyone, you will get a very different set of ratings after a few years, based on whether you are calculating ratings every year, every six months, every quarter, or every month. You might think that the more frequent cycles would actually result in more accurate FIDE ratings, but that is actually not at all true.
The Chessmetrics and Professional ratings are relatively unaffected by how frequently the ratings are calculated. It can only help to calculate ratings very frequently, because that way you get more up-to-date ratings. The Chessmetrics and Professional ratings differ significantly, however, in how far back they look while considering what games to use for the rating calculation. The Professional ratings always look back exactly 100 games, whether those games were played in the past five months or the past five years. Further, the more recent games are much more heavily weighted, so that half of your Professional rating is actually based on just your past thirty rated games. The Professional rating calculations don't care what a player's previous rating was; the entire rating comes from those 100 games. The Chessmetrics ratings, on the other hand, always look back exactly a year, whether that year includes zero games or 200 games. Of course, it will put correspondingly more emphasis on the past year's results, based on how many games were played. It is a matter of preference whether you think your "recent" results are best represented by a fixed time period, or a fixed number of games (that go back however far is necessary in order to reach the prescribed number of games).
Another serious flaw in the FIDE and Professional ratings is that they do not provide any statement about how accurate the ratings are. In Elo's book from a quarter-century ago, he provides a small table of numbers describing what the expected error would be in the ratings, for several different quantities of career games played (that table is the source of the "provisional until 30 career games" rule), but that is simply based on theoretical considerations; there is no empirical evidence to support Elo's assertion that those errors have any correspondence to reality. That approach also suggests that the accuracy of a player's rating is always increasing, as long as their number of career games keeps increasing. This is clearly wrong; if a player begins to play less frequently, then even though their career number of games is increasing, we become less and less sure about the accuracy of their rating. The Professional ratings are at least accompanied by a "variance", but that is simply a measure of how stable the player's performance rating tends to be in individual games; it says nothing about the accuracy of the ratings.
On the other hand, every Chessmetrics rating is accompanied by a corresponding +/- value, which represents the standard error (standard deviation) of the rating. Players can only qualify for the world ranking list if their +/- value is small enough to indicate a "significant" rating. A rating is an estimate of what the player's level of performance currently is, and the +/- value indicates the standard error of that estimate.
Another important drawback to the FIDE and Professional rating systems is that of inflation/deflation. This phenomenon has been widely studied, and it is clear that there has been considerable inflation in the FIDE ratings in the past decades. For instance, in the early 1970's Bobby Fischer's rating peaked at 2780, and Fischer's domination of his peers was far greater than the current domination of Vladimir Kramnik and Viswanathan Anand, both of whom have surpassed Fischer's 2780 mark in the past year. Any list of the highest-category-ever tournaments will invariably list only tournaments from the past five or ten years, also due to the rating inflation at the top. It is impossible to meaningfully compare FIDE ratings that are even five years apart, let alone ten or twenty. The Professional ratings have not been around nearly as long as the FIDE ratings, so it is not clear to what degree the inflation is occurring. However, I am not aware of any corrections for inflation/deflation in the Professional calculations, and since it is an ongoing performance rating calculation, it seems likely that there is nothing anchoring the average ratings to a particular standard.
On the other hand, the Chessmetrics ratings have been carefully adjusted in a serious attempt to eliminate any inflation or deflation. A rating of 2700 or 2500 should mean approximately the same thing in 2001 that it did in 1901. To learn more about my corrections for inflation, read the section lower down about inflation. This correction enables the comparison of ratings across eras. Of course, a rating always indicates the level of dominance of a particular player against contemporary peers; it says nothing about whether the player is stronger/weaker in their actual technical chess skill than a player far removed from them in time. So while we cannot say that Bobby Fischer in the early 1970's or Jose Capablanca in the early 1920's were the "strongest" players of all time, we can say with a certain amount of confidence that they were the two most dominant players of all time. That is the extent of what these ratings can tell us.
And, of course, the biggest flaw in the FIDE and Professional ratings is that they don't go far enough back in time. Elo's historical calculations and graphs are simply too coarse to be of any real use, and even the official FIDE ratings are of limited availability in the 1970's. Further, the FIDE ratings since 1980 were only calculated twice a year (until very recently), which is simply not frequent enough. The monthly Professional ratings are indeed more frequent, but they go back less than a decade.
My Chessmetrics ratings, on the other hand, are currently calculated weekly, and the monthly calculations go all the way back to 1980, and only the pre-1950 ratings are done as infrequently as once per year. But the ratings go all the way back to 1851, so for historical analysis it seems clear that the Chessmetrics ratings are far more useful than the FIDE or Professional ones, as long as you trust the accuracy of the Chessmetrics ratings.
Is there any reason why you shouldn't trust the accuracy of the Chessmetrics ratings? I'd love to say that they are perfect, but of course they are not. The biggest criticism of the ratings has to be that the source of games is not as cleanly defined as it is for FIDE (I don't know what source of games is used for the Professional ratings). I have not excluded rapid or blitz games, or even casual games, simply because there is no easy way to tell from a PGN game whether it should count as a "rated" game. Although I have invested considerable time working on the accuracy of the game results, I have not omitted any games due to the conditions under which they were played.
Now, even though my ratings do include all games rather than just regulation-time-control "serious" games, remember that those ratings do nevertheless outperform the FIDE ratings in their accuracy at predicting the outcomes of games. That fact goes a long way toward justifying the inclusion of those other games, but nevertheless it would be great if the 1.8 million games in my database could be somehow pared down to only "serious" games. I simply don't have the resources to do that, and I'm not convinced that such an action would necessarily improve the accuracy of the ratings themselves. It might, and then again it might not.
Not only does my game collection include too many games, you might just as well say that it includes too few games. Because I need up-to-date ratings for the purposes of my statistical analysis, I elected to use the TWIC games as my source for the past 2.5 years. This necessarily means that many games are excluded that would normally be included in a huge database like the ChessBase Mega Database. If that database were more timely, then perhaps I could use it, but instead I am almost forced to use the TWIC games, which could conceivably raise questions about the accuracy of the ratings in the past couple of years, for people who don't have all their games included in TWIC. Mark Crowther's opinion was that the TWIC approach should work well at least for the top 50. I know that when the next version of the big ChessBase database comes out, I can use it to plug some of the gaps, but that is a secondary concern right now. I apologize to anyone whose recent games are not included as a result of this decision, but I'll do what I can to remedy this situation, and I urge all tournament directors to make their games available to TWIC.
In addition, it is very difficult to get a "perfect" set of games that were played many decades ago. I worked very hard to get an accurate set of games (even those where we only know the result and not the moves; sometimes we don't even know who had the first move) up through 1880, but after that point it just became too difficult to keep up with the expanding tournament scene, and so there could easily be missing games, especially for tournaments which didn't manage to preserve the entire gamescores.
Finally, it will always be true that somewhere out there is a slightly better formula. I know that my ratings work better than the FIDE ones, but of course that doesn't mean that the Chessmetrics rating formula is the "best" one. I have tried to optimize it, based on the accumulated evidence of more than a million chess games, but it is almost certain that there is a better formula than the one I currently use. Nevertheless, it's the best formula I could find, and I did try a large number of other alternatives.
The Statistical Theory Behind the Ratings
The formula is based upon considerable empirical chess data from a very large database of historical games. The statistical theory behind the formula is based upon the Method of Maximum Likelihood and its application to certain variables which are assumed to follow normal distributions, those variables being:
(a) the error of a rating estimate; and
(b) the observed difference between a player's true rating and their performance rating over a subsequent time period (usually a year).
There is of course no abstract reason why those variables must follow a normal distribution (although (b) is a trinomial distribution which for more than a few games should indeed follow a normal distribution), but experience predicts that they would follow a normal distribution, and the empirical data seems to indicate strong agreement. Using that empirical data, I have created formulas which estimate the variance of those two variables listed above. The variance of (a) is based on the number of games played in recent years leading up to the calculation of the rating, and the variance of (b) is based on the number of games played during that year. In both cases, the formula actually uses the inverse square root of that number of games, since the statistical theory suggests that the variance typically would be proportional to that inverse square root.
The Method of Maximum Likelihood requires an "a priori", or "prior" distribution, as well as a "posterior" distribution. In the specific case of rating calculations, the prior distribution describes our estimate of a player's true level of skill, exactly a year ago. The mean of this distribution is the actual calculated Chessmetrics rating a year ago, and the variance is based upon the quantity of games played, leading up to that calculation. The posterior distribution represents a performance rating, namely the observed performance level of the player during the year in question. The mean of that distribution is the player's true level of skill a year ago, and the variance is based upon the quantity of games played during the subsequent year.
When you use the Method of Maximum Likelihood, you consider many different guesses for what the player's true level of skill was a year ago. Certain guesses are more likely than others; the most promising guess is that their calculated rating a year ago was exactly right, but of course it is quite likely that there was a certain amount of error in that rating estimate; probably the player's true level of skill was either underrated or overrated by that calculation.
For each guess under consideration, you first assume that the guess was exactly right, and then see what the chance would be of the player actually scoring what they really did score. The "likelihood" is then calculated as the probability of your original guess (as to the player's true skill over the past year) being right, times the probability (assuming that the guess was correct) of the player's actual results.
Let's try a small example to illustrate how this works. A hypothetical Player X has a rating of 2400, with a particular uncertainty associated with that rating. To keep it simple, let's say that there is one chance in two that Player X's true level of skill is actually 2400, one chance in five that Player X's true level of skill is actually 2500, and one chance in a hundred that Player X's true level of skill is actually 2600. Then Player X plays fifteen more games, with a performance rating of 2600, and the big question is how we revise Player X's rating. Is it still near 2400, is it near 2600, or is it somewhere in the middle?
Let's further pretend that if a player has a true rating of 2400, then they have one chance in fifty of scoring a performance rating of 2600 in fifteen games. And if they have a true rating of 2500, then they have one chance in ten of scoring a performance rating of 2500 in fifteen games. Finally, if they have a true rating of 2600, maybe there is one chance in three of scoring a performance rating of 2600 in fifteen games. These are all hypothetical numbers, of course; the real trick is to figure out what the actual numbers should be!
Using these simple numbers, though, we can calculate the "likelihood" of a particular rating estimate as the product of those two chances. The chance of Player X's original "true rating" being 2400 (one in two) times the chance of a 2600 performance rating in fifteen games by a 2400-rated player (one in fifty) gives an overall "likelihood" of one in hundred that their "true rating" really is 2400. The same calculation gives a likelihood of one in fifty for a 2500 rating, and a likelihood of one in three hundred for a 2600 rating. Thus, in this very simplistic example, our "most likely" estimate of the player's true skill is 2500, since that one has the greatest likelihood of being true (.02 vs. 01 or .003). And so, even though our previous guess of the player's true skill was 2400, the evidence of those fifteen subsequent games leads us to re-evaluate our current estimate of the player's true skill, to 2500.
This approach provides a middle ground between the conservative FIDE ratings, which will always be too slow to react to a drastic change in a player's ability, and the sensitive Professional ratings, which place no emphasis at all on a player's prior rating, looking only at a weighted performance rating that may overstate whether a player really has improved as much as their recent results would indicate.
Now, of course, there are more than just three possible "true ratings"; there are infinitely many, and this means you have to deal with probability densities rather than actual probabilities, and those densities are based on the density of a normal variable, which is a pretty ugly exponential formula. However, it all has a happy ending. It turns out that if your prior distribution is normally-distributed, and your posterior distribution is normally-distributed, then rather than maximizing the likelihood, you can instead maximize the logarithm of the likelihood, which lets you cancel out all of the ugly exponential terms. Also, the logarithm of "X times Y" is the logarithm of X plus the logarithm of Y, and it works far better to take the derivative of a sum than it does to take the derivative of a product, especially when you are going to be solving for one of your variables. Further, since you are maximizing it, you need only take the derivative of the log-likelihood function, with respect to the player's "true" rating. This lets you zero out several terms (that are not related to the player's "true" rating). By setting the derivative equal to zero and solving for the "true" rating, you get a very simple formula, which turns out to be a simple weighted average of the previous rating with the observed performance rating, with the weights being the variances of (b) and (a), respectively. Since (a) and (b) were defined many paragraphs ago, let me state them again:
(a) the error of a rating estimate; and
(b) the observed difference between a player's true rating and their performance rating over a subsequent time period (usually a year).
As long as you're still with me after all of that math, let me point out one more thing. The Professional ratings are just a special case of the more general equation. If you assume that the variance of (b) is zero, then your resultant rating will be exactly equal to the observed performance rating, and that's how the Professional ratings work. So, the Professional ratings assume that if your true rating is 2383 over a particular time period, you will always score an exact weighted performance rating of 2383 over a hundred games during that time period. That is clearly not true; even a thousand games is probably not enough to ensure such accuracy. The variance of (b) is definitely nonzero. So, if the Professional ratings were truly an attempt to estimate, as accurately as possible, a player's true level of skill, some weight needed to be given to what their rating was originally, since that does provide some useful information. However, perhaps the Professional ratings are simply intended to be an accurate measure of a player's recent results, rather than an estimate of how good a player really is.
Another possibility is that my statistics are flawed and that the Professional ratings actually are a great way to estimate a player's true skill. The real proof, of course, would come from comparing whether the Professional ratings work as well as the Chessmetrics ratings at predicting the results of future games. I would love to perform such an analysis, but unfortunately I have been unable to obtain a satisfactory set of historical Professional rating lists, or specific definition of how the details of the calculations work (so I could do it myself). Specifically, I don't understand how provisional players enter the list (since at the start they won't have 100 games played, and they won't be part of the original basis calculations); my inquiries to Vladimir Dvorkovich and to Ken Thompson have been unanswered. Mark Glickman, inventor of the Glicko rating system, has been very helpful in general, but couldn't help me out in this particular case.
The FIDE ratings, on the other hand, are far more available than the Professional ratings, allowing me to check whether I was really improving on the FIDE approach, or whether I was out of my league in my attempts to find a better approach. I can now confidently say that the Chessmetrics ratings work better than the FIDE ratings at predicting the results of future games, and thus the Chessmetrics ratings are more accurate than the FIDE ratings at estimating the true level of skill of chess players.
Still not convinced? Want to see the numbers? In order to keep myself honest, I decided that my process would be to use all games up through 1994 to develop my rating formulas, and then I would use the games of 1995 and 1996 to test whether the formulas really worked better. Otherwise, if I used all games through 2001 to develop my formulas, and then used some of those same games to compare rating systems, it wouldn't be fair to the FIDE system, since my formulas would already be optimized for those same games. So, I pretended that I had invented everything in 1994, and had then spent 1995 and 1996 checking to make sure that I had really improved on the Elo formulas. I didn't want the cutoff times to be much later than 1996, since my switchover to using TWIC games (rather than ChessBase) might influence the results.
This test was successful; the Chessmetrics ratings consistently outperformed the FIDE ratings, month after month after month. I can't provide the full details right now, though I promise to put them up on the site soon. I was hoping to include the Professional ratings in the mix before doing a full-blown analysis, but for now the only Professional ratings I have access to are the monthly top-fifty lists as published by Mark Crowther in his weekly TWIC issues. So perhaps we can only make conclusions about how the rating systems work among top-50 players. I did do an analysis of FIDE vs. Professional a year ago, using those top-50 lists, and found that the Professional ratings did no better than the FIDE ratings at predicting the results of future games, and maybe even a little worse than the FIDE ratings. The FIDE ratings still work quite well, and not really that much worse than my Chessmetrics ratings, but they are demonstrably inferior.
Correction for inflation/deflation
The final topic to be covered is that of rating inflation. Let me digress for a moment. If we wanted to compare the performance of today's golfers with past historical great golfers, we can do that, because it is easy to measure the absolute performance of a golfer. The same argument applies even more strongly to individual sports such as swimming or high-jumping or javelin throwing. There is still room to argue about whether the performances of today's swimmers are more impressive than the performances of past greats (who didn't have the benefits of today's training methods, or whatever), but there can be no doubt that today's top athletes swim faster, jump higher, and throw further than any of their predecessors.
Do today's top chess players play better than any of their predecessors? That question is harder to answer objectively, without an absolute standard to measure against like we have in track and field. Chess players compete against other chess players, and the average chess performance hasn't changed in centuries; it's still a 50% score. In the same way, we can't measure objectively the relative performance of Barry Bonds vs. Babe Ruth, or Muhammad Ali vs. Joe Louis, or Michael Jordan's Chicago Bulls against Bill Russell's Boston Celtics. All we can do is measure the degree to which they dominated their contemporaries. The same goes for trying to compare Garry Kasparov to Bobby Fischer to Jose Capablanca to Wilhelm Steinitz. If we had only had the foresight to lock Bobby Fischer in a room in 1972 so he could play thousands of games against an incredible supercomputer running the state-of-the-art computer chess program, we could drag that same computer out of mothballs today and begin to make progress on this question. We could emulate that same computer program on a Palm Pilot and pit it against Garry Kasparov, and then maybe we could start to say something about who was truly stronger, although there are huge problems with even that approach, since players learn about their opponents during competition, and presumably each player would win their final 1,000 games against that computer opponent.
To continue this ridiculous discussion a few sentences longer, we do have a special advantage in chess in that we have a near-perfect record of Bobby Fischer's performance in 1970-2, and the same to varying degrees for Garry Kasparov in 1999 and Jose Capablanca in 1922 and Wilhelm Steinitz in 1878, since we have the moves of all of their games; we just don't have the skills yet to construct an objective way for a computer to analyze whose technical play was truly "strongest". We have to resort to human analysis of their play, and so we enter the realm of subjectivity, which is probably where this question belongs anyway, given the undeniable human element whenever a human plays a game of chess.
Nevertheless, it is possible to measure (in an objective way) a player's performance against contemporaries, allowing us to sort a list of players from strongest to weakest, and we can express the relative level of skill of two players in terms of a "rating difference", which has a well-established meaning today. However, even if we know that Player A is the top-rated player, and Player B is second, 40 points behind, and Player C is five points back of Player B, what ratings do we give them? Should Player A have a rating of 2800, and B 2760, and C 2755? Or should Player A get a rating of 2.80, or 28 million? It doesn't matter for the purposes of a single list, but when we try to measure how much one player dominated in 1958 against how much another player dominated in 1859, it would be great to have some sort of meaningful scale to allow that sort of comparison.
Unfortunately, the Elo scale itself doesn't have any safeguards built in to prevent rating inflation/deflation, and it is clear that the meaning of a 2700 rating (for instance) is different if you are talking about 1971 versus 2001. In 1971, a 2700-rated player would be extremely dominant, and almost certainly the strongest player in the world. In 2001, a 2700-rated player is not even in the top ten in the world, and almost certainly NOT the strongest player in the world.
My original approach to this problem was to adjust all of the ratings so that the #10-rated player in the world received a 2600 rating, for all of the lists from 1850 to 2001. This was an improvement on having no correction at all, but hardly an optimal one. Long ago, there would have been far fewer players within 200 points of the world champion than we have today, so a world champion would have been almost expected to be 200 points higher than the #10 player in the world, whereas today it would be almost unheard of. So the "#10 player is 2600" rule seems unfair to modern players, among other problems.
I still liked the idea of anchoring a specific rating to a particular world rank #, but it needed to vary across time to reflect the fact that there are many more players today than ever before. I eventually hit on the scheme (based on a suggestion from a reader of my original Chessmetrics site) of having the anchor world rank # depend upon the world's population at the time. The general rule is that for every 200 million people in the world, I will use one slot further down in the world rank # as my anchor. So if the world population were 2 billion, I would use the #10 player as my anchor, but in modern times as the world population neared 6 billion, I would eventually use the #30 slot as my anchor.
Does that mean that the anchor would always receive the same rating? No, since there is no guarantee that the spacing of players at the top should be directly related to the world population. Instead, I used the actual historical lists that I had already generated, as a guide for what the anchor slot's rating should be. I wanted the rating of the top players each year to be about the same (and I picked 2800 as the desirable rating for the #1 player), but I didn't want to follow that rule too closely, since then we would see Fischer and Capablanca and Steinitz and Kasparov all having the same 2800 rating, which would be kind of pointless.
I plotted the gap between #1 and #5 across 150 years, and fit that data to a straight line or at most a simple curve. Then I did the same thing for the gap between #5 and #10, and for #10 and #12, and in fact for many different gaps involving top-30 players. Using this data, I could predict what the gap should be between #1 and #12 on a particular year, by adding the three predicted gaps together. Let's say that gap was 140 points. Then, if the anchor for that year was the #12 slot (because the world population was about 2.4 billion), I could work backward from the desired goal of 2800 for the #1 player, and the predicted gap of 140 points, to arrive at anchor values for that year: I would add/subtract the exact number of rating points to give the #12 player a rating of 2660, and then everyone else on that list would get the same number of points added/subtracted to their rating, so that the relative differences between ratings stayed the same. The top player would be measured against other historical greats by looking at whether this top player really did rate 140 points higher than the #12 player, or whether they managed a gap higher or lower than the prediction.
By fitting the data to simple lines and curves, I hoped to capture overall trends among top players, while still allowing the #1 players across eras to differentiate themselves. There were still potential pitfalls. For instance, what should we do if there happened to be a big clump of players right above or right below the anchor slot? Answer: use a weighted average of the five players centered on the anchor slot, instead of just the one player. Another big hurdle was what to do when a few top players retired simultaneously (or showed up simultaneously), and suddenly threw off the ranks. Answer: at the end, go through a few iterations of trying to minimize the overall change in ratings for players between their previous and subsequent ratings, possibly causing entire lists to move up or down a significant amount, though I only considered players whose world rank hadn't changed by more than a few slots. Today the anchor slot is fixed at #30, and the rating given to the #30 slot is slowly increasing, to reflect the fact that as the population of chess players increases, we should see slightly more clumping at the top, so the gap between #1 and #30 should be slowly decreasing.
Conclusions, and Looking Ahead
So, there you have it; I don't have too much more to say at this point, other than the fact that I expect to eventually revise much or all of the above process thanks to my receiving constructive criticism from all of you who are reading this. Interestingly enough, this effort was more of a means to an end, rather than an end in itself; I wanted a sound way to arrive at the error of a rating estimate, to allow me to do better statistical analysis than what I have done so far in my articles for KasparovChess.com. Now I have those error values, so I can move on to interesting topics like the true odds of players' winning the FIDE knockout championships, or what the best candidates' system is for determining a challenger to the world champion, and things like that. However, I also have great hopes for improving this site. Here are my immediate plans for the future of this project:
(1) The whole point of switching over to the TWIC games was so I could have very up-to-date ratings. Currently the ratings only go up through September 10th, 2001 (since that was the last TWIC issue I had imported before I did my final run of rating calculations), but as soon as I get some time I plan to implement a process where I can bring in a TWIC issue each week and somehow update the site with the latest ratings. I'm still working on the finer points of some of that. Currently the site uses static HTML pages, but my plan is to switch over to dynamically-generated ASP pages from my database, as soon as I know that I won't be hurting the performance of the site by doing that.
(2) I know that there are still some embarrassing parts of my rating system. It still has Gata Kamsky in the top 15, even though he seems quite retired, so perhaps I need to ease my rules about what "retired" means. Also, if you look at some of the age graphs for very young players who didn't play very many games, you can see an interesting cyclical effect which seems to indicate problems in my calculations of provisional ratings. Finally, I have a correction which I apply to provisionally-rated players to reflect the "regression to the mean" effect: the fact that their calculated ratings are probably too far away from the average rating. That one seems to work, but probably I should do a similar thing for players who are no longer provisional, but who nevertheless have uncertain ratings due to not playing very much. This would probably help in forcing down the ratings of older or semi-retired top players. It would be great if I could somehow adjust my algorithm to take care of these problems. Probably I'll incorporate these changes the next time I modify the set of games used (like if I do more cleanup work on the 19th century games) and have to rerun the entire ratings calculations from 1850 again.
(3) I know that my decision to use TWIC games will mean that I lose a lot of events, so many of the 13,000+ players will have some games excluded and their ratings will be correspondingly more uncertain. In addition, at the other end of the continuum, I know that my game collection is imperfect, especially in the pre-1920 era. I tried to do a really good job through 1880, but I'm sure that errors slipped through, and who knows what it's like between 1880 and 1920? Ideally, I would go through Jeremy Gaige's landmark books on tournament crosstables, and find the next-best-thing for match records (which are not included in Gaige's books, I believe), and manually enter all of the results (or at least check them against the ChessBase data and add missing games, which is basically what I did through 1880). At this point, I've done the best I could do in my limited spare time away from the rest of my life; maybe some of you can help me out somehow. If Gaige's books were computerized, that would be a great first step. By the way, while I'm on the subject of Jeremy Gaige, I would like to mention that I made use of his excellent book "Chess Personalia: A Biobibliography" to enter birth and death dates, as well as consistent spellings, accent marks, etc., for everyone who had ever been in the top 100 of one of my date lists. That book is about 15 years old, so I know that there are many players who must have died since then, and many spellings that I don't have the ability to check against a master source. I apologize to anyone who I have wrong data for, and I tried to do the accent marks like Gaige did, though there were some special characters that my database wouldn't accept.
(4, 5, 6, ...) I want to add the ability to get graphical plots for any player, rather than just the 99 players who ever been in the top 5 in the world. I want to include more top lists, like who have the best peak ratings, or the best peak-five-year ratings. I want to include nationalities so my friends from Denmark and Sweden and Iceland who are looking for their countrymen can see interesting lists limited only to a particular country. I want to show the analysis which compares the FIDE, Professional, and Chessmetrics rating performance. I want to add another dimension of "openings" to all of this. I want to add the ability to drill down to individual lists of games for each player, and to view those games. I want to add my past and future articles about various statistical topics. I want to add the ability for a user to generate their own graphs of historical data about requested players. We’ll see whether I manage to do any of those!
Thanks for reading all of this, and I hope you enjoy my site.
 - Jeff Sonas
Back to top
| |
| |